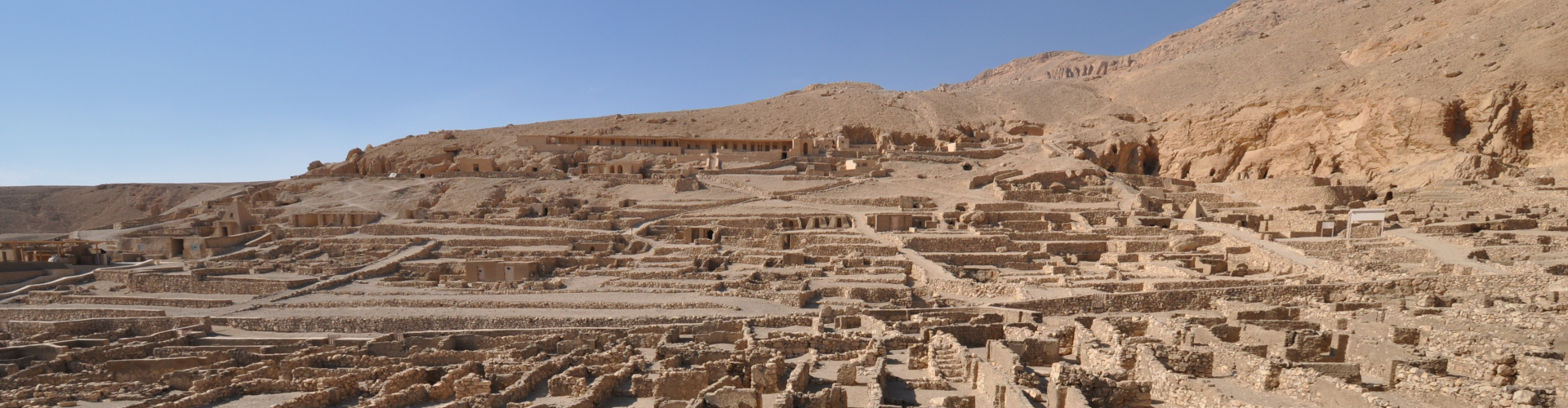
|
|
|
Selected multitemporal satellite images have been chosen for each site.
A geodatabase of the looted traces already identified and mapped has been created to train the Deep Neural Network developed.
The metadata used to label pit-polygons in the images have been carefully defined. Each feature class contains information about the singles looting pits, their shape/type and their reliability.
The developed Machine Learning Model is a Supervised Deep Neural Network for Change Detection on Satellite Imagery. This model analyses images of the same scene at two different time steps and automatically segments the newly appeared looting pits.
Most of the training dataset is used for optimising the Deep Neural Network performance by learning from its prediction errors. While the rest is used to estimate how the model performance generalizes on “new data”.
A ground truthing campaign has been carried out in Aswan (Egypt), where 129 features of the curated geodatabase were verified. The change detection model has been re-trained removing the misidentified looting pits.
Risk Maps have been elaborated and created for each case study, relying on the density and distance of the looting pits, and the proximity to known archaeological areas.