The project is working toward the creation of the first publicly available multi-modal dataset of labelled archaeological sites and toward solving the problem of the non-standardisation of performance metrics. This benchmark dataset will contain Sentinel 2 multi-spectral images and LiDAR data of the archaeological landscape of Aquileia (Italy), a major city of the Roman Empire.
State-of-the-art object detection and semantic segmentation methods are being explored to establish how the granularity of the detection affects the quality of the prediction from an archaeological point of view. To assess the performance of the different models, a set of different metrics will be introduced with the collaboration of landscape archaeologists in order to set a first reference standard for promoting objective cross-study measurements. Archaeological ground-truthing will be undertaken in the case study area to verify the predicted results provided by the developed AI methods. The data collected during the ground-truthing activities will be used to improve the identification performance of the proposed methods.
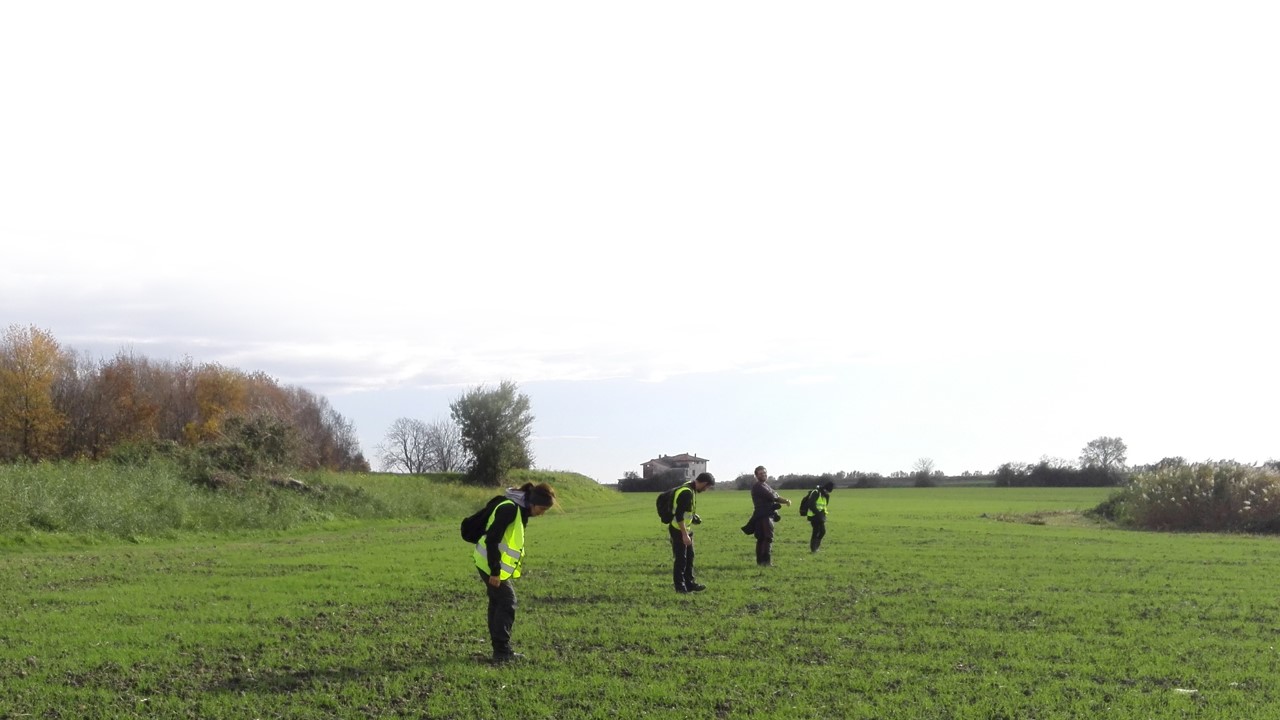